Google Discover: Key Insights On Various Topics
Is there a hidden world of data influencing your decisions, shaping outcomes in ways you may not even realize? Understanding the Variance Inflation Factor (VIF) is key to unlocking insights hidden within statistical models and ensuring your analyses are robust and reliable.
The world of data analysis is complex, a landscape filled with intricate relationships and subtle influences. For those navigating this terrain, the Variance Inflation Factor (VIF) emerges as a crucial tool, a compass guiding us through the potential pitfalls of multicollinearity. Multicollinearity, the unwelcome presence of highly correlated predictor variables in a regression model, can wreak havoc on the accuracy and interpretability of our results. It distorts the estimates of regression coefficients, making it difficult to ascertain the true impact of each variable on the outcome we are trying to understand. The VIF, a measure of how much the variance of an estimated regression coefficient increases because of collinearity, provides a means to identify and quantify this threat. A high VIF value signals that a predictor variable is highly correlated with other predictors, potentially leading to unstable and unreliable model results. Consequently, it's crucial for anyone working with data to be aware of VIF, how to calculate it, and how to interpret its significance in the context of their models.
Consider the case of emergency services, where data-driven decisions are critical. Imagine analyzing factors that predict response times for ambulances. Variables such as the number of emergency calls, traffic conditions, and distance to the scene are likely to be correlated. If the ambulances location is close to other variables then its response time also influenced by other variables like traffic conditions and calls. High multicollinearity, if present, could cloud the true influence of each of these factors on response times, potentially leading to inaccurate resource allocation or deployment strategies. Addressing multicollinearity, perhaps by removing or combining correlated variables, is vital for ensuring the integrity of the model and the effectiveness of emergency services.
The term "Best 9vids.com porn videos, top rated incest taboo scenes" represents a stark contrast to this world, highlighting a completely different sphere. It stands in stark contrast to the professional and data-driven world of analysis and planning. It speaks to an entirely different audience and interest, a realm of adult entertainment that is entirely separate from the pursuit of statistical accuracy and predictive power. The association is, at best, jarring and highlights the broad and often disparate nature of online content.
The use of "Watch family xxx movies and shorties for free every day!" further emphasizes this contrast. The focus here is on readily available, freely accessible content. This kind of material is often promoted in ways that generate traffic, sometimes creating echo chambers. This is the opposite of the type of rigorous analysis required to understand and interpret the VIF.
In a completely different vein, the mention of "Insurance provider for emergency service organizations, volunteer fire departments, ambulance, rescue squads and 911 centers in united states and canada," brings us back to the realm of serious service. This suggests an organization that provides financial security and support for those involved in the crucial work of helping others during emergencies. This sector also relies heavily on understanding risk and data to provide coverage.
In the fast-paced digital age, the phrase "Make your link do more" becomes critical. Businesses and individuals alike are constantly searching for efficient ways to manage their online presence and connect with an audience. This sentiment emphasizes the importance of optimization and maximizing the impact of one's digital footprint. The focus here is on increasing productivity and streamlining information delivery.
Similarly, "One link to share everything you create, curate and sell across ig, tiktok and more" reflects the realities of today's digital landscape. Platforms like Instagram and TikTok have become vital hubs for content creators and businesses, requiring efficient distribution of content across multiple channels. This phrase indicates a need for integration and streamlining of online marketing.
The core of the matter, however, returns to the statistical concept of multicollinearity and the Variance Inflation Factor (VIF). The following provides a focused overview of the VIF, its role, and how to interpret its outcomes.
Variance Inflation Factor (VIF) - Understanding the Core Concept:
The Variance Inflation Factor (VIF) is a statistical measure used in regression analysis to quantify the severity of multicollinearity in a multiple regression model. Multicollinearity refers to the situation where two or more predictor variables in a regression model are highly correlated. This can cause problems in interpreting the model coefficients, as the model may struggle to determine the unique effect of each predictor. VIF helps us identify and quantify this problem. The formula to calculate VIF for a predictor variable is:VIF = 1 / (1 - R-squared)Where R-squared is the coefficient of determination obtained by regressing the predictor variable on all other predictor variables in the model. A high VIF (typically above 5 or 10) indicates high multicollinearity, suggesting that the predictor variable is highly correlated with other predictors and that the model's estimates may be unstable and unreliable.
Interpreting VIF Values:
- VIF = 1: No multicollinearity. The predictor variable is not correlated with any other predictors in the model.
- 1 < VIF < 5: Moderate multicollinearity. The predictor variable is moderately correlated with other predictors, but the multicollinearity may not be severe enough to cause major problems.
- 5 < VIF < 10: High multicollinearity. The predictor variable is highly correlated with other predictors, and the multicollinearity may be significant enough to affect the model's estimates.
- VIF > 10: Very high multicollinearity. The predictor variable is very highly correlated with other predictors, and multicollinearity is likely to cause serious problems in the model.
Consequences of High Multicollinearity:
- Unstable coefficient estimates: Small changes in the data can lead to large changes in the estimated coefficients.
- Inflated standard errors: The standard errors of the coefficients are inflated, making it more difficult to determine the statistical significance of the predictors.
- Difficulty in interpreting coefficients: It can be hard to distinguish the individual effects of correlated predictors.
- Reduced model accuracy: Multicollinearity can reduce the model's ability to predict future outcomes.
Addressing Multicollinearity:
- Remove highly correlated predictors: If two predictors are highly correlated, remove one from the model.
- Combine predictors: Create a new predictor variable by combining the correlated predictors (e.g., by averaging them).
- Collect more data: More data can sometimes reduce the impact of multicollinearity.
- Use regularization techniques: Techniques like ridge regression or lasso regression can help to reduce the impact of multicollinearity.
The Vanessa K. Connection:
The "Driver training refresher to meet vanessa k." and "Free driver training program on vfis university is a free training program developed by vfis to assist emergency services personnel comply with the vanessa k." and "Free emergency services training act of 2005.follow the instructions contained within this course to obtain the vanessa k." sections refer to the Vanessa K. Standard. The details are critical in the emergency services field. The Vanessa K. Standard is a set of requirements. The training and compliance are essential for emergency personnel in order to uphold safety and professionalism. The training programs likely provide the knowledge and skills necessary to satisfy this standard, ensuring that individuals are prepared for their roles and can respond to various situations effectively and safely.
Practical Application - Multicollinearity in Action:
Consider a regression model predicting house prices. Predictor variables might include: square footage, number of bedrooms, number of bathrooms, and location. It is highly likely the number of bedrooms and the square footage of a house are highly correlated. A high VIF for these variables would alert the data analyst to the potential problem of multicollinearity. If the model shows high VIF, the analyst would need to consider either removing one of the variables or creating a combined variable, such as "size". Doing so can improve the reliability of the model and allow for more accurate estimations.
Tools and Resources:
Statistical software such as R, Python (with libraries like scikit-learn and statsmodels), and SPSS provides tools for calculating VIF. These tools allow analysts to quickly assess the degree of multicollinearity in their models and to identify potential problems. The availability of these tools highlights the importance of understanding and addressing multicollinearity in any statistical analysis.
Beyond the Data:
The various elements present in this collection of phrases, from the highly specific details regarding the Vanessa K. training requirements to the more general emphasis on online marketing (make your link do more), reveal the diverse nature of the information one encounters daily. This can often seem overwhelming and sometimes requires careful discernment to determine the validity or applicability of each piece of information. By understanding the core ideas present, one can assess the information accurately and make informed decisions.
Aspect | Details |
---|---|
Name | Variance Inflation Factor (VIF) |
Category | Statistical Measure |
Purpose | To quantify the severity of multicollinearity in a multiple regression model. |
Multicollinearity | The situation where two or more predictor variables in a regression model are highly correlated. |
Formula | VIF = 1 / (1 - R-squared), where R-squared is the coefficient of determination obtained by regressing the predictor variable on all other predictor variables. |
Interpretation | A high VIF (typically above 5 or 10) indicates high multicollinearity. |
Consequences of High Multicollinearity | Unstable coefficient estimates, inflated standard errors, difficulty interpreting coefficients, and reduced model accuracy. |
Remedies for Multicollinearity | Remove highly correlated predictors, combine predictors, collect more data, or use regularization techniques. |
Key Concept | The degree to which a predictor variable is correlated with other predictors in a model and how this correlation affects the variance of the parameter estimates. |
Related Areas | Regression analysis, Statistics, Data analysis, Machine learning |
Software Tools for Calculation | R, Python (with libraries like scikit-learn and statsmodels), and SPSS. |
Importance | Critical for ensuring the reliability and interpretability of regression models. |
Use Cases | Predicting house prices, analyzing sales data, modeling medical outcomes, and many more. |
Ultimately, understanding the Variance Inflation Factor is not just about statistics; it is about building robust, reliable models, ensuring your data tells a truthful story, and empowering you to make more informed decisions, no matter your field of expertise.
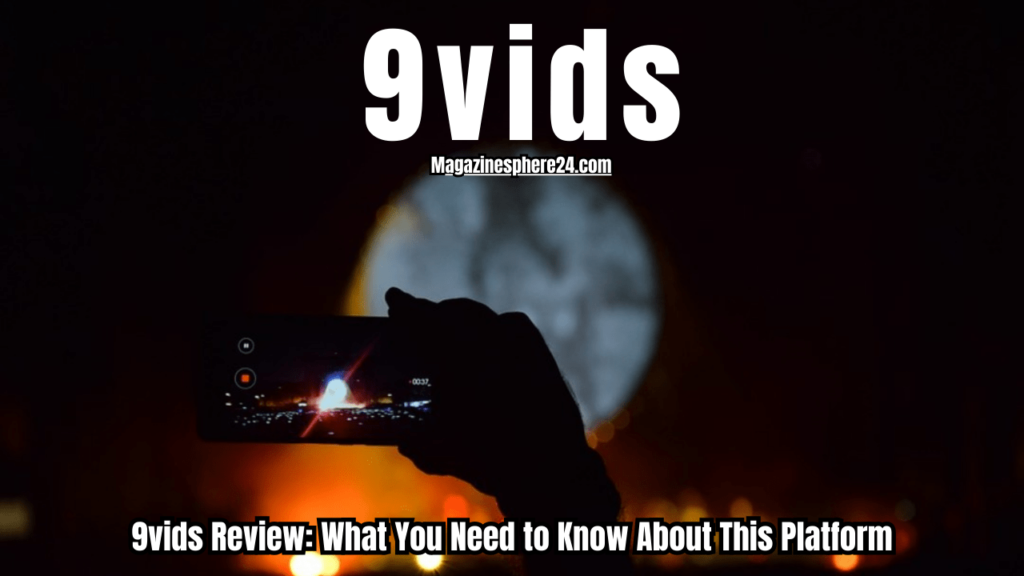
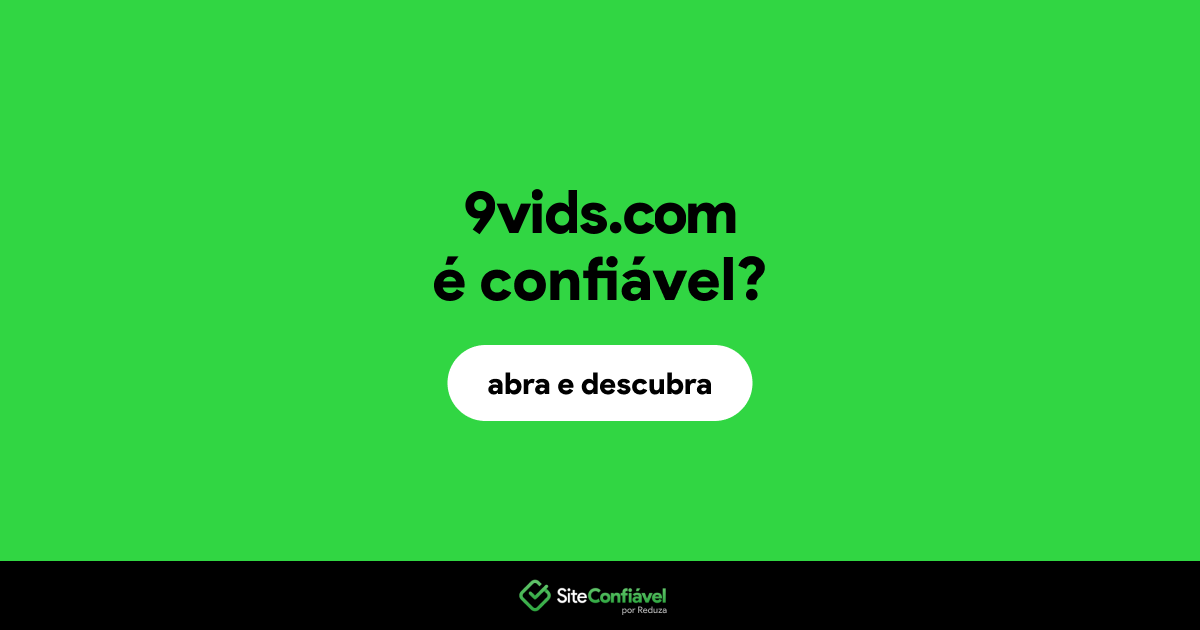

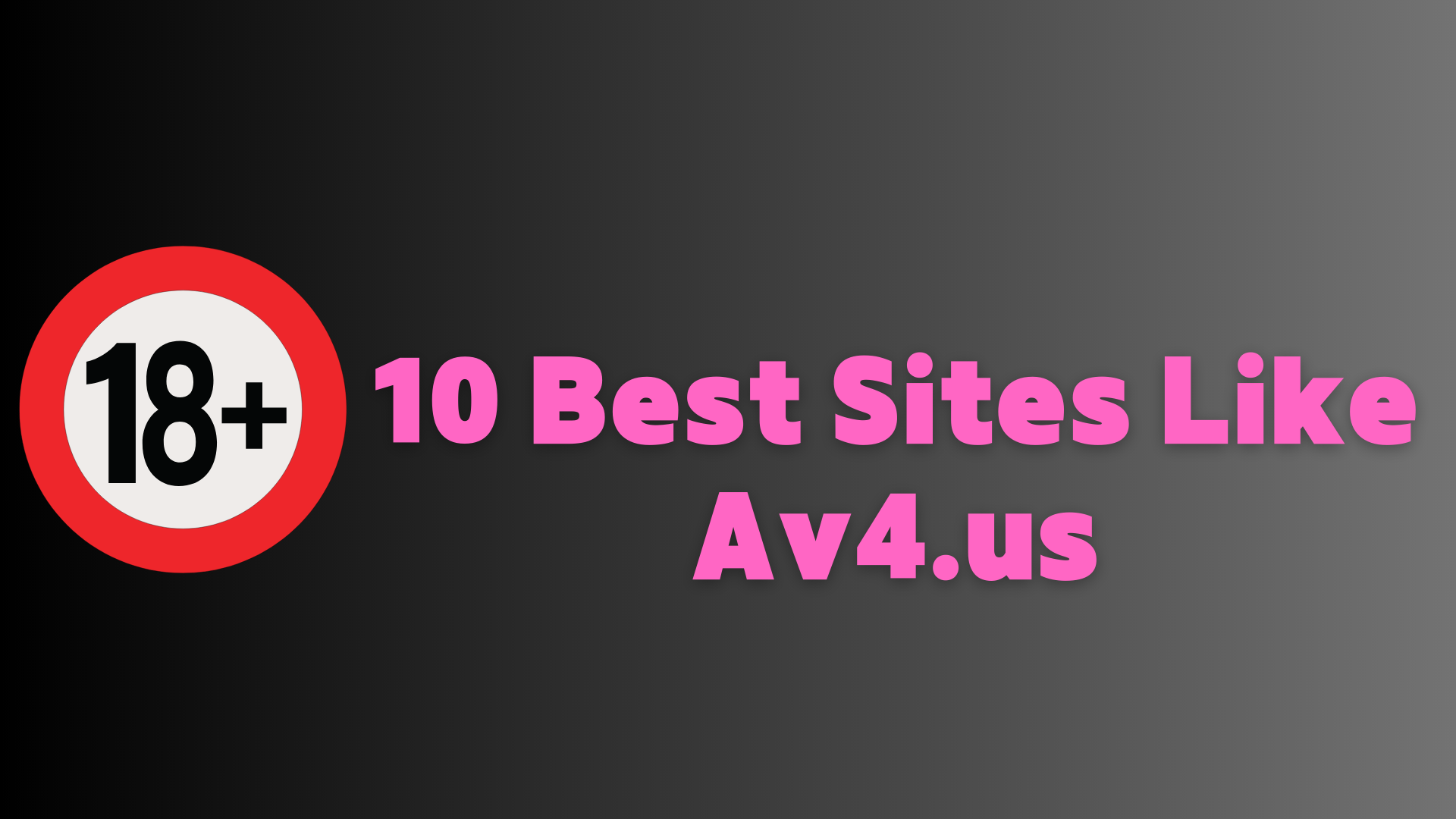